As businesses continue to grow and expand, the need for robust, data-driven decision-making increases. To meet this demand, many organizations build and grow their analytics stacks—integrating different tools, platforms, and technologies to enhance data collection, analysis, and reporting. While this process might seem straightforward, several hidden challenges can make growing an analytics stack more difficult than expected.
Let’s explore the unexpected challenges you’ll face when growing your analytics stack and how to avoid the common pitfalls that turn promising data initiatives into expensive headaches.
High Hidden Costs
On the surface, a business might see the price of purchasing tools or paying for subscription fees as the direct cost, but this is far from the total cost of ownership.
Let’s start with the fact that many analytics platforms have ongoing operational costs that can balloon over time. While you might start with a few core tools, as the business scales and new requirements emerge, you find yourself layering on specialized tools—each with its own cost structure. There’s the initial licensing fee, but then there are integration costs, data storage fees, training costs, and sometimes custom development needed to make everything work smoothly.
Huge Time Drain
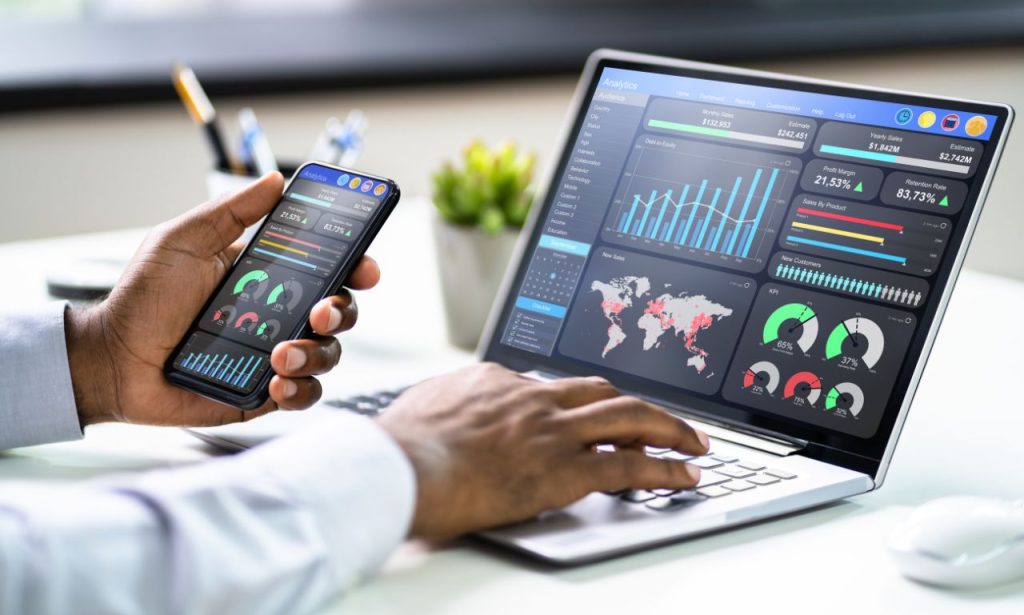
I was astounded by how long it took to integrate and synchronise everything when scaling my own analytics setup for a project. Every new tool must be configured, set up, and frequently troubleshooted. The proper KPIs must be set, and tools must be connected to ensure data moves between them seamlessly.
As data teams grow, they inevitably spend more time keeping the lights on rather than focusing on strategic projects. In many cases, teams become so bogged down with the sheer volume of operational tasks—such as ensuring data quality, updating tool versions, or responding to system alerts—that they lose sight of the bigger picture: using data to drive business outcomes.
Credibility
With more tools in your tech stack, data discrepancies are more likely to occur. This becomes particularly problematic if your analytics stack is not well-integrated and well-governed.
For instance, data may be stored in multiple silos or exported from several different sources. Departments may become confused and draw incorrect conclusions due to inconsistent data definitions or misaligned data models. I can’t count the number of times I’ve encountered circumstances where teams’ reporting didn’t match, only to discover that the disparity was caused by various data definitions being used in different systems.
For this reason, data governance procedures and tools are essential in large-scale analytics settings. Without them, preserving the credibility and integrity of data can become extremely difficult.
ROI
Return on investment (ROI) is perhaps one of the most talked-about metrics when it comes to analytics tools, but measuring the ROI of your analytics stack can be deceptively difficult. Many organizations struggle to quantify the true value of the data and insights provided by their tools.
It’s tempting to think about ROI regarding the direct cost vs. direct benefit—for instance, does tool X lead to more sales or conversions? However, the real benefits of a well-functioning analytics stack aren’t always immediately measurable. Even more accurate forecasting, enhanced operational efficiency, or better decision-making may be observed, but it may be challenging to directly link these advancements to a particular instrument or procedure.
Furthermore, expanding an analytics stack usually necessitates initial infrastructure, talent, and technology expenditures. It’s important to have a long-term view and measure ROI in the context of sustained growth rather than quick wins, even though businesses are frequently optimistic about the immediate return they’ll see.
Overwhelm
It’s easy to get caught up in the excitement of new analytics capabilities. But as your tool stack grows, you may face tool overload. There’s a tendency to keep adding new features, more specialized tools, and broader capabilities in hopes of solving every possible problem. This only leads to a bloated tech stack that’s difficult to manage and maintain.
Take it from me—more tools don’t always mean better results. I added several advanced analytics tools a year ago, thinking they would give us deeper insights into customer behavior. In reality, each tool had a unique interface, and integrating them into a cohesive workflow became so complicated that it led to inefficiencies rather than improvements.
It can be difficult to balance having a comprehensive analytics stack and not overcomplicating the environment. Sometimes, having fewer but better tools is the smarter choice.
Data Team Becomes an Ops Function
As your analytics stack grows, your data team may spend more time on operations than strategy. The more tools you introduce, the more work it takes to maintain them. At some point, data teams are no longer viewed as strategic partners in decision-making but as support staff responsible for fixing errors, monitoring system health, and maintaining the stack.
For instance, you might find that your data team spends the majority of its time ensuring data flows correctly across systems, resolving issues with tool integrations, or dealing with vendor issues, leaving little room for them to provide the actionable insights the business really needs.
This shift from strategy to operations can be detrimental to an organization’s long-term growth, as it means your analytics team is too busy putting out fires to focus on delivering high-impact insights.
Inflated Wants
Departments may become enthused about the “shiny” new features that analytics tools offer and want to incorporate them into the stack because they believe they will provide instant benefits. But value isn’t always correlated with features. Unnecessary features or tool capabilities that are rarely used or don’t support company objectives can eventually weigh down a company. Significant tool bloat may result, raising operational expenses, complexity, and data governance issues without increasing business value.
Tool Inflation
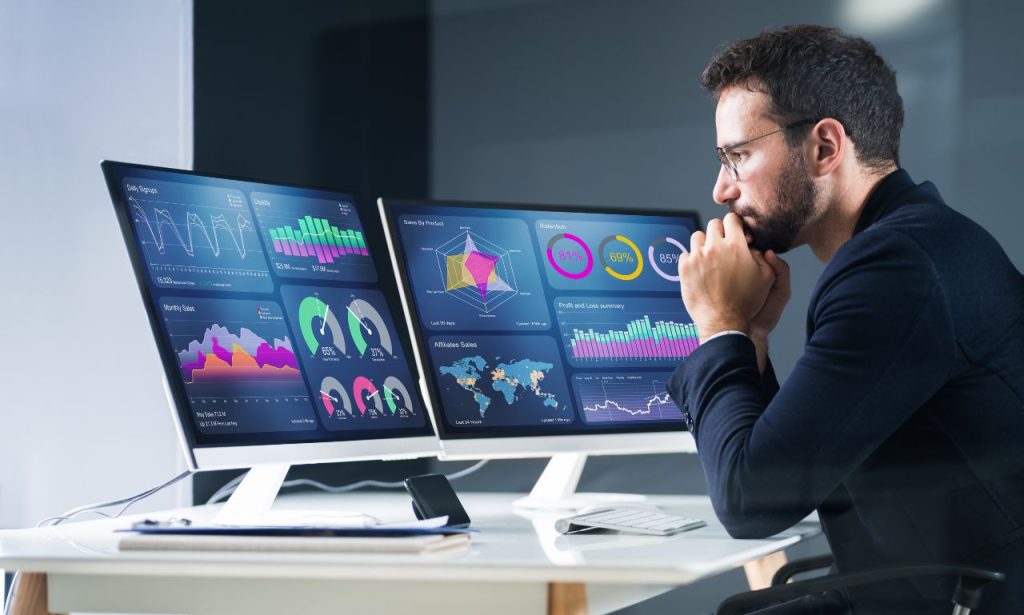
The analytics ecosystem constantly evolves, and each tool promises to make your life easier. But soon, you find yourself caught in a tool inflation trap—where your stack is overrun with specialized tools that don’t integrate well, have overlapping functionality, or simply aren’t being used to their full potential.
Conclusion
Growing your analytics stack comes with its fair share of challenges, from hidden costs and inflated tool stacks to the overwhelming complexity of integration. But it’s not all doom and gloom. You can avoid many of these pitfalls by carefully planning your analytics stack, focusing on long-term sustainability, and constantly evaluating whether each tool adds value.
The key takeaway is that growth isn’t just about adding more tools or technologies—it’s about making smarter decisions that align with business goals, keeping costs in check, and ensuring your team isn’t overwhelmed.
ALSO READ :
FAQs
A: Focus on integrating only the tools that align with your current business goals and scale gradually. Avoid chasing after every shiny new feature, and make sure tools integrate well with your existing systems.
A: ROI can be tricky, but focus on the long-term benefits, such as improved decision-making, efficiency, and strategic alignment, rather than immediate results. Measure success using metrics like operational efficiency, user satisfaction, and the impact on key business objectives.
A: Always account for both direct and indirect costs. Implement cost-containment strategies such as consumption-based pricing models and periodically reassess your tool stack to eliminate redundant tools.